Nillion disruptive innovation: opening up a new era of AI privacy computing
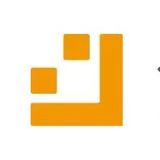
Reprinted from jinse
02/19/2025·3MAuthor: Teng Yan & 0xAce; Translated by: Golden Finance xiaozou
In the field of artificial intelligence, privacy is no longer an option - it is about life and death.
Nillion is a decentralized infrastructure designed to manage privacy-sensitive data for applications such as AI and private DeFi. The team has reached cooperation with Virtuals, NEAR, Aptos, Arbitrum and others. The Nillion ecosystem is rising, so let’s explore this article in depth.
本文摘要:
-
Nillion is redefining privacy protection computing—a key link in the absence of next-generation AI.
-
隐私不再是可选项。 AI agents need access to sensitive data to work, but today's infrastructure forces users to choose between privacy and practicality.
-
盲计算改变了这一现状。 Nillion supports computing on encrypted data without exposing data, thus solving AI trust issues.
-
The Nillion network architecture consists of two key components, each with a unique and important role: the coordination layer (nilChain) and Petnet (Privacy Enhancement Technology Network).
-
多层隐私保护。 Instead of relying on a single approach, Nillion seamlessly integrates MPC, FHE, ZKP, and TEE into a privacy stack.
-
A strong team (including Uber’s founding engineer and senior cryptography expert) and strong financial support have raised $50 million from top investors.
-
NIL的代币经济学设计旨在推动采用。 NIL will power the network to ensure operational security while driving destruction-based demand as usage scales grow.
For decades, we have dreamed of having a personal AI assistant.
From Iron Man's JARVIS managing every aspect of Tony Stark's life (suspended him occasionally), to the computer in Star Trek's exact knowledge that Picard likes to drink too much hot tea (always hot), to Her Samantha in the middle – She not only sorted out Theodore’s inbox, but also philosophically thought about existing problems when she fell in love.
They reflect a common truth: being a human being is tiring.
JARVIS不仅操作托尼·斯塔克的战衣。 He also schedules meetings, orders groceries, pays bills, and may suggest that meetings at 3 a.m. are not good ideas for good health.
When we see this, we think: Yes, that's it.我需要这个。
We keep inventing these fictional assistants because deep down we all desire that someone—or something—can relieve the ruthless spiritual burden of modern life.
An assistant who can remember what we forget, discover patterns we ignore, and may even prevent us from sending the message that we will 100% regret the next day at 2am.
1. Privacy Issues
But reality is different from fiction: JARVIS knows everything about Tony Stark because, well, it's a movie.
Movies never faced the privacy nightmare of AI fully accessing every corner of someone’s life.
What happens in the real world? This is a trillion-dollar challenge waiting to be resolved.
At present, AI agents are still in the early stages of development - posting on Twitter, creating anime avatars, making some jokes. But this is changing rapidly. We are on the verge of greater change.
To really help us, these AI agents need access to our most sensitive information.
Think about what information JARVIS actually knows about Tony:
-
Complete financial status – every account, every investment, every secret project.
-
Relationships – from conversations with his wife Pepper Potts to communication with the Avengers and enemies.
-
Health data – complete biometrics, medical history, shrapnel near the heart.
-
Professional Secrets – Not only Iron Man’s Suit, but also all the intellectual property rights of Stark Industries.
Now imagine giving this level of access to today's AI agents. Will you feel at ease? This is not a hypothetical wish list – it is the type of data that AI really needs to be our digital representation.
An AI financial advisor who doesn't know your complete financial situation is just a beautified calculator.
An AI health assistant without your complete medical records? Just a WebMD with a better UI.
In order for AI to change from something novel to necessity, we must solve the privacy problem.
2. Dilemma
Today’s AI infrastructure often forces us into a dilemma:
1) Give up privacy for practicality
Leave all data to centralized AI companies;
Believe that they will keep the data safe (Pray);
Accepting your data may be used to train the model.
2) Keep privacy but sacrifice practicality
Stick to the use of local AI models with limited functionality;
Missed advanced features;
Accept mediocre performance.
Take the AI health assistant for cancer patients as an example.
To provide meaningful support, it requires access to your full medical record, genetic test results, current treatment options, medication schedules, side effects reports, and even your private conversation about your true feelings.
Without strong privacy guarantees, you are actually broadcasting your most private health struggles to unknown servers and employees.
Imagine your most vulnerable moments—your fears, your pain, your treatment decisions—handled in plain text on the company’s infrastructure.
The real problem is how AI systems process data.
When you use tools like ChatGPT or Claude, the following happens: Your data is sent to their server → processed in plaintext → and may be stored for future model training.
Yes, they have privacy policies and security measures. But at the end of the day, you still rely on trust.
This may be acceptable when the AI is just writing emails or generating cute cat pictures. But as AI becomes more personalized and powerful, this privacy crisis becomes about survival.
3. New paradigm requirements
The solution is more than just a better encryption or a stricter privacy policy. It's like installing a lock on a house without walls.
We need to fundamentally reimagine how AI systems handle sensitive data. The infrastructure we need can:
-
Compute on encrypted data without exposing data
-
Provide encryption privacy protection
-
Spread trust so that no single entity holds all power
The next wave of AI isn't just about smarter models or faster performance. It will be about trust.
Can we build a system that is powerful enough to process our most sensitive data and trustworthy enough to let us share that data?
Can we create privacy infrastructure that makes real-life JARVIS possible?
These questions will define the next chapter in AI development.
Nillion 's story begins here.
4. Nillion=blind calculation
Nillion is creating a completely new paradigm called " blind computing ."
Through it, you can have all the capabilities of an advanced AI system without exposing your sensitive data. It’s not just encrypted storage, but actually doing the encrypted data, which remains private from beginning to end – even for the infrastructure that handles it.
But to understand why this is revolutionary, we need to understand the shortcomings of current privacy solutions.
(1)超越传统隐私
Many existing privacy solutions are like locking data in a safe – it is safe when it is still, but when you want to use it you have to take it out.
Every time you calculate something, you expose the data. It's like taking them through crowded streets every time you need to process sensitive files.
This "decryption-computing-encryption" process creates a window of vulnerabilities that experienced attackers can exploit. Even with strong encryption, your data will be exposed during the calculation process, making it vulnerable to memory crawling, side channel attacks, and other vulnerabilities.
Nillion's blind calculation allows data to be calculated in an encrypted state.
(2) The power of coordination
The current field of privacy agreements is decentralized and specialized. There is no shortage of solutions, but each has its limitations:
-
Multi-party computing (MPC) is good at safe distributed computing, but it is difficult to scale.
-
Fully homomorphic encryption (FHE) supports calculations on encrypted data, but is slow and costly.
-
Zero Knowledge Proof (ZKP) provides powerful verification without exposing data, but is limited by computing power and bandwidth.
-
Trusted Execution Environment (TEE) provides practical security, but requires hardware trust assumptions.
Most projects choose a method and optimize around their strengths while accepting their limitations. It's like choosing an instrument and trying to play the whole symphony.
Nillion is not content with solo. It is to conduct the entire orchestra.
These privacy enhancement technologies (PETs) work together to create a larger privacy solution than the sum of each part.
Let's dive into how Nillion can achieve this technically.
5. Underlying network architecture
The Nillion network architecture consists of two key components, each with a unique and important role:
-
Coordination layer (nilChain)
-
Petnet (Privacy Enhanced Technology Network)
Together, they implement blind calculations.
(1) Coordination layer
The coordination layer built on the Cosmos SDK, called nilChain, acts as the control center of the network. It does not process private data or runs calculations. Instead, it ensures that the network runs efficiently and securely, like a high-performance operating system that manages processes and resource allocation.
Why choose Cosmos SDK?
The Cosmos SDK was chosen because of its modular architecture, high scalability and interoperability. Cosmos is designed for sovereignty, allowing nilChain to run independently while benefiting from cross-chain compatibility through cross-chain communications (IBC) .
In fact, this means that Nillion can interact safely and efficiently with other blockchain ecosystems without relying on traditional bridges, which are often a security risk.
The role of nilChain
Although built on Cosmos, nilChain is deliberately kept lightweight. It does not store or process any sensitive data. Instead, it focuses on:
-
Payment processing—NilChain handles economic logic every time someone uses Nillion’s computing service—like a decentralized billing system.
-
Rewards and staking – Node operators pledge tokens for participation; in return, they receive fees for their honest work. Malicious nodes may lose their stake.
-
Network Security - nilChain also maintains the global ledger of node stakes, reputation and tasks.
-
Coordination – nilChain coordinates which node clusters handle specific computing, ensure load balancing and assigns tasks to nodes with appropriate capabilities (e.g., clusters that specialize in large-scale MPCs).
This minimalist approach is crucial because it enables the coordination layer to focus entirely on coordination while leaving complex privacy-protected calculations to Petnet.
As of now, nilChain is running in the test network environment , and the main network is expected to be online this month.
(2) Petnet
While the coordination layer manages the operation of the network, Petnet (Privacy Enhanced Technology Network) is the real wonder of Nillion.
This is a network of dedicated privacy processors, each contributing to a larger system that can perform calculations on encrypted data without seeing actual information.
Petnet combines different privacy technologies through a dedicated clustering system. Rather than forcing each node to process each type of computation, nodes are grouped into clusters that specifically handle specific tasks.
Cluster architecture: scale and specialization
This clustering approach solves one of the fundamental challenges in privacy- protected computing: balancing security and performance.
Some calculations require maximum security and can tolerate slower speeds, while others require lightning-fast processing with real-time applications. By making the cluster specialized, Nillion achieves both.
Think of these clusters as specialized departments in the company. Just like you won't let your accounting team handle product design, you don't want every node to try to handle every type of calculation. Some clusters may focus on:
-
Maximum security for highly sensitive data
-
Real-time processing of time-critical applications
-
Optimal performance for specific types of computing
6. Privacy Technology
Petnet combines three cutting-edge privacy enhancement technologies (PETs) to provide higher levels of security and efficiency.
(1) Multi-party computing (MPC)
MPC allows multiple parties to jointly calculate a function while keeping their input private. Nillion's custom MPC protocol is designed to eliminate the communication overhead of traditional MPCs, which makes typical MPCs slower.
-
How it works – not a single entity processes sensitive data, but a fragment of encrypted information held by multiple nodes (called "particles"). Each node processes only its data shares, ensuring that no single entity can reconstruct the original input.
-
Secret Sharing and Blinding – Use Shamir's Secret Sharing and one-time mask to split private input data into multiple encrypted shares to prevent unauthorized access.
-
Local computing without interaction - nodes independently compute without communication, preventing data leakage during the process.
-
Reconstruction and Verification – After the calculation is completed, the node combines its results shares to reconstruct the final output, ensuring correctness without exposing sensitive inputs.
By removing communications during the computing process, Nillion's MPC achieves speed and scalability that was previously considered unrealistic.
(2) Fully homomorphic encryption (FHE)
Fully homomorphic encryption (FHE) allows calculations on encrypted data without decryption. Although FHE is computationally expensive, Nillion selectively integrates FHE to play its role where it is most valuable.
-
Why choose FHE? ——Unlike MPCs, MPCs split data between nodes, while FHE supports computing directly on encrypted data without secret sharing.
-
FHE as a supplementary module - Nillion uses FHE when secret sharing is not feasible, such as financial transaction calculations that must be kept end-to-end.
-
MPC + FHE – In some cases, FHE is used in conjunction with MPC, allowing the system to encrypt data before it is shared across nodes. This ensures that the data remains encrypted at every stage of processing.
The network also integrates preprocessing technology to reduce the complexity of homomorphic operations, making FHE feasible in actual deployments.
(3) Trusted Execution Environment (TEEs)
TEEs provide hardware-based isolation for safely performing sensitive computing within a processor. Nillion selectively utilizes TEEs through its nilTEE framework.
-
How TEEs works - TEEs are secure areas within the processor that are used to isolate running code and prevent sensitive data from being exposed to a wider range of systems.
-
AI Model Execution – TEEs support secure AI model inference without exposing model weights or inputting data.
-
Threshold Signature and Authentication – for secure encrypted signatures and authentication.
-
Short-term secure computing – Nillion uses TEEs only in performance-critical tasks, ensuring long-term data protection remains decentralized.
By integrating TEEs with MPC and FHE, Nillion balances performance, security, and decentralization, ensuring that computing remains private while delivering high-speed results.
7. Make privacy practical: Development stack
All these complex privacy techniques will be useless if developers cannot easily use them.
That's why Nillion created a suite of development tools that abstract the complexity of privacy-protecting computing.
The current toolkit includes the following components:
(1) nilVM:
A privacy-first virtual machine that changes the way developers write secure applications.
Using a Python-based language called Nada , developers can write code that feels like normal programming—but behind the scenes, it compiles into a privacy-protected operation. Think of it as a general-purpose translator that converts regular instructions into secure multi-party computing without the need for developers to worry about cryptography.
VMs support basic operations and more complex tasks such as threshold signatures of messages, making them particularly suitable for financial applications that need to process sensitive data while maintaining privacy.
The module is accessible via the SecretSigning SDK, which utilizes nilVM's encryption capabilities for threshold signature and authentication workflows.
(2) nilDB:
nilDB makes processing encrypted data as simple as using a regular database. Unlike traditional systems, data in traditional systems must be decrypted for querying or analysis, while nilDB keeps all information encrypted while still allowing complex operations .
It splits data into multiple nodes using a combination of secret sharing and MPC, ensuring that no single node sees the complete information.
Developers can write familiar SQL-like queries, and nilDB automatically handles all privacy protection operations.
This makes it ideal for medical systems that require analyzing patient data while maintaining strict privacy compliance, or financial services that require detection of fraud patterns without exposing personal transaction details.
The system even supports secure multi-party analytics, allowing organizations to gain insights from a combined dataset without having to disclose their underlying data to each other. It can be accessed through the following SDK:
-
SecretVault : A vault-like service for securely uploading and managing encrypted data.
-
SecretDataAnalytics : allows authorized query or analysis of encrypted data sets without exposing the underlying information.
(3) nilAI:
A set of privacy technologies focused on AI to make private machine learning practical. It contains three key components:
-
AIVM (AI Virtual Machine) : Nillion-based MPC technology and integrated with Meta's CrypTen framework, enabling AI models to process data while keeping the model and data private.
-
nada-AI : Provides an interface similar to PyTorch, allowing developers to easily handle privacy protection AI models. You can train neural networks, run inference, and handle machine learning tasks using familiar patterns.
-
nilTEE : uses a trusted execution environment for secure AI processing, especially suitable for running large language models in private.
The system uses technologies such as discrete wavelet transformation (study with Meta's AI research team) to accelerate encrypted AI operations. This enables practical performance for running complex AI workloads—from personalized AI assistants that keep user data private to enterprise AI systems that need to keep data confidential while delivering real-time results.
From request to result: end-to-end process
Now that we have seen how nilChain, Petnet and blind modules work together, let's look at typical user interactions—from storing data to running AI models.
Here is a brief process:
1) The user starts the user or application to interact with Nillion's SecretSDK or API (e.g., "Save this file safely" or "Run this function on my data").
2) The client prepares the SDK to encrypt or secretly share the data so that no party can view it in full. This may involve MPC shares or processing encapsulated data for TEE.
3) Coordinate requests to nilChain through nilChain , nilChain records requests, handles $NIL payments, and assigns tasks to specialized clusters in Petnet. Different clusters may focus on storage (nilDB), computing (nilVM), or AI inference (nilAI).
4) Blind execution in Petnet
nilDB (Storage) : Data shares are distributed in nodes in the cluster.
nilVM (Computation) : Node runs the MPC protocol on its data shares—no single node sees the full input.
nilAI (AI Inference) : TEEs or hybrid FHE/MPC methods ensure that no raw data or model weights can be seen even the node operator. At this stage, each cluster node only processes encrypted or masked data.
5) Results and settlement
Cluster completion results (e.g., storing shares, returning calculation results, or sending encrypted AI responses).
nilChain confirms completion, releases payment to participating nodes, and handles any punishment for malicious behavior. The user ends up decrypting the result locally (if needed).
This workflow provides end-to-end privacy and verifiability, nilChain coordinates tasks, and Petnet executes securely behind the scenes.
8、Nillion技术实践
Nillion technology is already handling large amounts of workloads and has gained widespread attention from developers. Fortunately, it 's not just a theory.
Developer adoption is growing rapidly, with the Nillion SDK being downloaded 961 times in just two months - an early signal to the platform's emerging developer ecosystem.
By providing the foundation for privacy-protecting computing and storage, Nillion is unlocking new product and service categories in multiple fields.
Here are some examples:
(1) Private AI Agent
As mentioned earlier, the next wave of AI agents will need to process increasingly sensitive data in order to be truly useful. Nillion's blind computing infrastructure enables AI agents to process private information without exposing it:
· Skillful AI has implemented private retrieval enhancement generation (RAG) using nilVM, allowing users to leverage sensitive documents during inference while minimizing data exposure.
· Rainfall has built a self-owned AI platform focusing on collective social intelligence, while protecting user privacy through security weight aggregation.
Verida is developing a personalized AI chatbot based on sensitive user messages training, using nilDB for secure storage.
This transition from entertainment-oriented AI agents to practical-oriented AI agents usually requires bulletproof privacy guarantees that can only be provided by blind computing. As AI agents evolve to dealing with investment, governance and negotiations, Nillion's infrastructure has become crucial.
(2) Medical Application
Healthcare is another huge opportunity for blind computing, and the AI medical data market is expected to grow from US$32.3 billion in 2024 to US$208.2 billion in 2030, achieving a 524% growth.
·Safe research collaboration: Healthcare institutions can analyze patient data across regions without exposing sensitive information. · Diagnosis of privacy protection: AI-based diagnostic tools can maintain complete confidentiality while processing patient data. · Compliant data sharing: collaborate while meeting strict medical privacy regulations.
HealthBlocks demonstrates this potential, allowing users to collect data from different sources while maintaining ownership and control, and then use Nillion to generate insights for third parties without revealing specific details.
(3) DeFi and sensitive data At present, the transparency of DeFi puts traders at the risk of MEV attacks, preemptive trading and market manipulation. Kayra, a decentralized dark pool DEX, solves this problem by using Nillion's blind computing to enable private institutional transactions. · Secret Order Book: Orders are encrypted and processed privately to prevent market influence. · Blind Computation Match (nilVM): Orders are submitted in encrypted form and processed using Nillion's MPC node. Each node performs calculations on the data fragment without seeing the complete order, ensuring confidentiality. When a match is found, only the final transaction details are decrypted for settlement, preventing leakage at each stage. · MEV and pre-emptive transaction protection: Orders remain private before execution, ensuring fair pricing.
By using Nillion, Kayra provides trustless privacy, making DeFi feasible for institutions and large-value traders. If successful, it could have a revolutionary impact on private transactions on chain.
Other DeFi applications using Nillion include: ChooseK is developing an order book platform that supports private DeFi products. Kagami creates a trading strategy engine in nilVM for encrypted financial policies.
With the World Economic Forum estimated $867 trillion in flows into DeFi, privacy-protected infrastructure becomes critical for institutional adoption.
(4) Blockchain integration Nillion has also established strategic partnerships with major blockchain platforms to make blind computing accessible on these chains: · Arbitrum: Privacy tool to enhance Ethereum expansion · NEAR protocol: Privacy protection application development Aptos: A privacy-focused application infrastructure · Sei: Native integration of secure computing
These integrations allow smart contracts and users to leverage blind computing directly from their favorite chains, using native gas tokens – no new wallet required.
(5) The key partnerships that promote adoption are meaningless if no one uses them. Strategic partnerships are accelerating adoption of Nillion in multiple areas, with major collaborations demonstrating the diversity and potential of the platform. Collaboration with Meta’s AI research team has made breakthroughs in privacy-protected AI computing, while co-ownership with Virtuals Protocol’s AI agent and decentralized AI reasoning with Ritual demonstrates Nillion pushing boundaries in emerging fields ability.
Combining its strong technological foundation and growing ecosystem adoption, these strategic alliances allow Nillion to capture important value when privacy-protected computing becomes the necessary infrastructure.
9. NIL Token Economics
NIL tokens are the core of Nillion's blind computing network, both a practical token for the network and a governance mechanism. With a total supply of 1 billion, NIL aims to coordinate incentives from all network participants while achieving sustainable growth in the ecosystem.
Note: NIL tokens are not online at the time of writing.
(1) Supplier: Allocation and unlocking
Token Allocation Most of the token supply (45%) will be used in the community and R&D to continuously improve technology, showing concern for sustainable growth rather than short-term gains. The agreement also retains 7.5% of the total supply (75 million NIL) for Creation Airdrops to early supporters and builders , with the goal of those who make meaningful contributions to the development of the network.
Unlock timetable
Token releases will follow a planned unlocking schedule:
-
The initial circulating supply is approximately 13.9% (139.6 million tokens)
-
The main unlocking event began 6 months after the creation of the world, with supply reaching approximately 30%.
-
It gradually increased to about 48% in the 12th month after TGE
-
Long-term linear attribution of team and ecosystem allocation
The first year schedule is quite radical: after six months of no new tokens, supply jumped from about 14% to more than 30%, and then climbed to nearly 48% in the 12th month. Since most of these first year unlocks come from investors and team allocations (there is always controversial on CT…), teams and communities drive strong network usage and create enough token demand to absorb increased supply is critical important.
(2) Demand side: Utility and governance
NIL plays a central role in protecting the network and providing access to its services:
Network access
-
Computation and storage operations: In the initial stage, users destroy NIL tokens based on a US dollar-denominated credit system. All Petnet operations are currently 100% destroyed.
-
Module-specific pricing: nilVM: fixed rate destruction for compute/storage operations, optional pre-destruction of tokens on a specific cluster to obtain credit to reduce latency; nilDB and nilAI: subscription-based model, requiring monthly destruction of NIL to access the API .
-
Predictable Cost: Operate non-transferable credit pricing in USD to ensure the stability of the company's cost.
Node stake
- Verifiers and Petnet Clusters: Node operators stake NIL to protect their roles and may face cuts due to malicious behavior. As the value of NIL grows, the network theoretically becomes safer with higher economic penalties.
Governance and resource allocation
-
Proposals and Votes: NIL holders affect protocol upgrades, fee structures and ecosystem funding.
-
Resource deployment: Governance sets parameters for community resource allocation.
10、供需结合
In the early stages, 100% of all Petnet operation expenses were destroyed, creating an important token destruction pool as network usage grew. Operational expenses are set through governance decisions and plan future transitions to permissionless, cluster-based pricing models.
This combination of predictable, dollar-denominated costs and full token destruction creates a clear and powerful connection between network adoption and token value. This is exactly what we like to see in token design.
Nillion’s token economics is built for long-term games. The system is designed to thrive as network adoption expands, relying on three key drivers of sustainability:
-
Use → Value: As network activity grows, more tokens are destroyed, driving NIL prices to rise.
-
Staking → Security: The rise in token value encourages node operators to pledge more tokens, increasing network security and flexibility.
-
Governance → Consistency: Token holders play a direct role in developing cyber policies to ensure that those with vested interests guide their development and long-term success.
If the network captures a small portion of the $270 billion cloud computing and storage market, the token destruction generated by network usage may quickly exceed the most radical unlocking schedule—promoting the long-term value creation of NIL tokens.
11. Team and Financing
Nillion has formed a truly world-class team that combines deep expertise in cryptography, distributed systems and product development.
The leadership team brings together veterans from Web2 and Web3:
-
Alex Page (CEO) comes from a traditional financial background, worked as a general partner at Hedera SPV and a banker at Goldman Sachs.
-
Andrew Masanto (CSO) is a serial entrepreneur with multiple exit experience and brings valuable ecosystem building experience as co-founder of Hedera and founding CMO of Reserve.
The technical foundation is laid by several key leaders:
-
Dr. Miguel de Vega serves as chief scientist and brings deep expertise in data optimization cryptography with 30 patents. His research background is crucial to Nillion's innovative approach to privacy enhancement technology.
-
Conrad Whelan is the founding CTO, leveraging his experience as a founding engineer at Uber to build scalable distributed systems. His experience building services to millions of users is particularly valuable because Nillion aims to be the core infrastructure.
We can at least see that the team is very strong and has been building together for many years. All signs point to a bright future.
In terms of financing, Nillion has received substantial financial support to achieve its vision.
They have raised $50 million from well-known crypto investors including Hack VC, Hashkey Capital, Distributed Global and Maelstrom. With an experienced team and a wealth of financial support, Nillion is ready to tackle the complex (and ambitious) challenge of building a privacy-protected computing infrastructure.
12. Our thoughts
(1) Orchestration is the real moat
Nillion's approach is to focus on orchestrating multiple privacy enhancement technologies (PETs) instead of betting all of its bets on a single approach. This strategy is smart, for two key reasons:
First, it creates selectivity. Different use cases have different privacy requirements and trade-offs. Some prioritize speed over maximum security, while others require regulatory compliance or specific encryption standards. By orchestrating multiple PETs, Nillion can provide the right tools for each job, rather than forcing everything through a one-size-fits-all solution. Secondly, it provides future guarantees for the platform. With new privacy technologies emerging (they will appear), Nillion can integrate them into its orchestration layer without breaking existing applications. This adaptability will be crucial as advances in quantum computing drive today's privacy technology.
Of course, it is not easy to build an effective orchestration layer. The challenge is to make it seamless for developers. If the experience is too complex or clumsy, Nillion's powerful solutions can be impressive in theory but not available in practice.
(2) Use privacy as infrastructure, not function
The conclusion after reading this article should be that Nillion positioned privacy as the infrastructure for next-generation computing . Think about how SSL/TLS becomes the default setting for network communication. We no longer talk about "encrypted websites" because encryption is how the network works. Nillion's bet is that blind calculations will follow the same trajectory. As AI agents become increasingly integrated into our lives and undertake increasingly sensitive tasks, privacy will no longer be optional—it will be a prerequisite. Just as no serious business today will launch a website without HTTPS, future applications will not be able to run without built-in privacy guarantees.
Timing will be the key. Entering too early, the market may not feel urgency yet. Entering too late, centralized solutions can be deeply rooted. Nillion's success will depend on entering the market at the right time —when privacy issues become so sharp that developers and businesses start actively seeking solutions. If they can seize this wave, Nillion has a real chance to make privacy-protected computing the default standard for next-generation applications.
13. Privacy Revival
Nillion is dealing with the biggest obstacle to AI: trust.
By seamlessly integrating cutting-edge privacy technologies with AI, Nillion has the potential to be the foundation of AI, just as SSL is to the network. If Nillion makes it powerful and easy to use, it will drive the next wave of AI adoption. High risk, but great opportunity. The privacy revolution has just begun. Let's wait and see!