Is DePAI the next encryption trend?
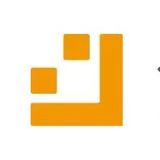
Reprinted from jinse
02/13/2025·3MTranslated by: Golden Finance xiaozou
DePAI (Decentralized Physical AI) is regarded by many cryptographers as the next major event (momentum) and is " one of the few areas that can use blockchain and encryption incentives to have a substantial impact on other technical fields." what is it? What are the innovations? What kind of potential does it have? Next, let’s find out.
Simply put, it is an innovative concept that combines decentralized physical infrastructure networks (DePINs) with artificial intelligence (AI) technology, coordinates physical hardware facilities of multiple individual units through blockchain technology to allow permissionlessness. , build and maintain infrastructure networks in a trustless and programmable way.
Messari analyst Dylan Bane supports DePAI on the X platform and publishes the following article:
Decentralized Physical Artificial Intelligence (DePAI) provides an alternative to centralized control of robotics and physical AI infrastructure stacks. DePAI is developing rapidly, from real-world data collection to physical artificial intelligence agent operating robots deployed by DePIN.
Nvidia CEO Jensen Huang said: " The 'ChatGPT moment' in the field of general robots is coming."
The digital age starts with hardware and gradually evolves into the invisible world of software. The era of artificial intelligence began with software, and now it is seeing the physical world as its ultimate challenge and cutting-edge field.
In a world where robots, cars, drones and bionics powered by autonomous physical artificial intelligence (Physical AI) are gradually replacing human labor, the issue of ownership of these machines has become a core social issue. Decentralized Physical Artificial Intelligence (DePAI) provides an important opportunity to establish Web3 physical AI before centralized players dominate.
The decentralized physical artificial intelligence (DePAI) infrastructure stack is developing rapidly. At this stage, the most active level is the data collection layer, which provides real-world data for training physical AI agents deployed on robots, while transmitting data in real time to navigate the environment and completing tasks.
Real-world data is the main bottleneck in training physical artificial intelligence (Physical AI). Although Nvidia's Omniverse and Cosmos provide a promising path through simulated environments, synthetic data is only part of the solution. Remote operation is also indispensable as real-world video data.
In the field of remote operations, FrodoBots is deploying low-cost sidewalk delivery robots worldwide using the Decentralized Physical Infrastructure Network (DePIN). This data collection method not only captures the complexity of human navigation decisions in the real environment, thereby generating high-value data sets, but also effectively overcomes the capital gap problem.
Decentralized Physical Infrastructure Network (DePIN) accelerates the deployment of data collection sensors and robots through its token-powered flywheel effect. For robotics companies seeking to accelerate sales and reduce capital expenditures (CapEx) and operating expenses (OpEx), DePIN offers significant practical advantages over traditional methods.
Decentralized Physical Artificial Intelligence (DePAI) can leverage real-world video data to train physical AI and build a shared spatial understanding of the world. Hivemapper and NATIX Network may be an important source of this data with their unique video datasets.
Decentralized Physical Artificial Intelligence (DePAI) can leverage real-world video data to train physical AI and build globally shared spatial understanding capabilities. With their unique video datasets, Hivemapper and NATIX Network are expected to be important data sources in this field. As Mason Nystrom pointed out, “Data is difficult to monetize at the individual level, but it is easy to convert value after aggregation.” Real-world data can be aggregated through the decentralized physical infrastructure network (DePIN), thus forming high-value Dataset. IoTeX's Quicksilver protocol realizes data aggregation across DePINs, while taking into account data verification and privacy protection, providing key technical support for this ecosystem.
The space intelligence/computing protocol is also committed to achieving spatial coordination and decentralized control of real-world 3D virtual twins through the decentralized physical infrastructure network (DePIN) and decentralized physical artificial intelligence (DePAI). The Posemesh protocol of Auki Network realizes real-time spatial perception capabilities while protecting privacy and decentralization, providing innovative technical solutions for this field.
The initial application of Physical AI Agents has also emerged. SAM is connected to Frodobots' fleet of robots all over the world and can infer geographical location. With the help of frameworks such as Quicksilver, artificial intelligence agents are expected to access data streams provided by the decentralized physical infrastructure network (DePIN) in real time in the future.
The most direct way to reach physical artificial intelligence is probably through investment-based decentralized autonomous organizations (DAOs).
XMAQUINA provides its members with access to physical artificial intelligence assets, including machine RWA, Decentralized Physical Infrastructure Network (DePIN) protocol, robotics companies, and intellectual property (IP), and is supported by internal research and development.
Crypto researcher DeFi Cheetah responded positively to Dylan Bane’s remarks about DePAI:
Decentralized physical artificial intelligence (DePAI) is the next major development direction in the field of encryption. Blockchain and encryption incentive mechanisms will empower spatial intelligence - that is, the ability of robots to perceive the environment, understand surrounding objects or structures in real time and respond effectively. This is one of the most challenging problems in the field of artificial intelligence robots. Our industry can help solve the most critical bottleneck in developing spatial intelligence - obtaining fine-grained, high-quality and continuously updated spatial data.
Achieve powerful spatial intelligence requires massive amounts of data that not only capture visual cues (such as colors and textures), but also include deep geometric contexts (such as polygons, point clouds, topological representations) and physical properties (angles, distances, frictions force, material type, etc.). While traditional 2D images or basic GPS coordinates have some value, they are often oversimplified for training advanced models designed to run in dynamic, complex, and unpredictable real-world environments.
● Complexity of 3D map construction
Projects such as Google Street View or dedicated LiDAR scanning can provide high-resolution 3D maps, but are expensive and the generated data sets are relatively sparse. For example, a high-resolution LiDAR device can cost more than $50,000, while a city-wide scan operation can easily cost hundreds of thousands of dollars. This cost complexity often leads to low update frequency, making maps obsolete within months.
● Limitations of centralized data pipelines
In many countries, most of the spatial data is controlled by state institutions or large enterprises. Since these centralized entities collect data only in specific regions, large areas around the world—especially rural or underdeveloped—are still in a state of unmapping or outdated data. In addition, proprietary data restrictions may lead to market fragmentation and hinder innovative research.
● Lack of 3D datasets
Although annotated 2D image datasets (such as ImageNet containing more than 14 million annotated images) have shown explosive growth, annotated 3D datasets are still scarce. Creating such datasets requires the combination of sensor fusion techniques such as LiDAR + Vision + IMU readings and a lot of manual annotations. This process is extremely time-consuming and expensive, which slows down the research and development process of robotics and machine learning applications.
Driven by the popularity of mobile devices, the crowdsourcing model recognizes that billions of smartphone and wearable devices worldwide can jointly provide massive amounts of location-based data. Modern smartphones are equipped with a variety of sensors—accelerometers, gyroscopes, magnetometers, cameras, GPS chips, etc.—those sensors can capture spatiotemporal data far beyond simple latitude and longitude. This model helps achieve the following goals:
● Real-time data collection
Imagine commuters capturing 3D scans of urban infrastructure during their daily commutes, or residents of remote villages record trails, building profiles and farmland boundaries with their mobile phone cameras alone. Over time, these seemingly tiny contributions will accumulate into a global integrated spatial database.
● Diversified environmental coverage
As mobile devices are nearly ubiquitous, their data naturally covers a wider range of geographical areas, topography and cultural environments. This geographical diversity is crucial for the robust AI model that must be learned to adapt to variable climates and community layouts.
● Democratization of data collection
By lowering the threshold for participation, the crowdsourcing model subverts the traditional centralized model. Individuals around the world can easily contribute data while sharing improvements in maps, navigation applications and AI innovations without the cost of costly large-scale data acquisition costs for a single entity.
As an incentive and verification layer, blockchain plays the following key roles:
● Trust and data integrity
Distributed ledger technology ensures that every contribution—whether it is a geotagged photogram, a small photogrammetry scan or a sensor log—is stored in a tamper-proof manner. Since each submitted data is hashed and recorded on a public or private blockchain, both users and researchers can trace the source and authenticity of spatial data.
● Tokenized incentive mechanism
Blockchain-based tokens can provide micro rewards based on the quality, quantity and relevance of submitted data. Contributors are compensated through smart contracts, and when the data meets certain criteria (such as clarity, geospatial accuracy, novelty), the smart contract automatically distributes tokens to participants. By providing fair and transparent incentives, the platform encourages continuous high-quality data contributions—a key requirement for building large-scale and maintained updated data sets.
● The open ecosystem of spatial data
Decentralized ecosystems are not susceptible to single-point failures or data monopoly. Tokens have spawned a micro-economic system that encourages diversified entities such as professional cartographers, AI labs, enthusiasts, startups and smartphone users to participate in collaboration, thereby enhancing the quantity and reliability of data flows.
Decentralized Physical Artificial Intelligence (DePAI) is one of the few areas that I think can have a substantial impact on other technology fields by leveraging blockchain and crypto incentives.