AiSci: On the commercialization of biopharmaceutical modality
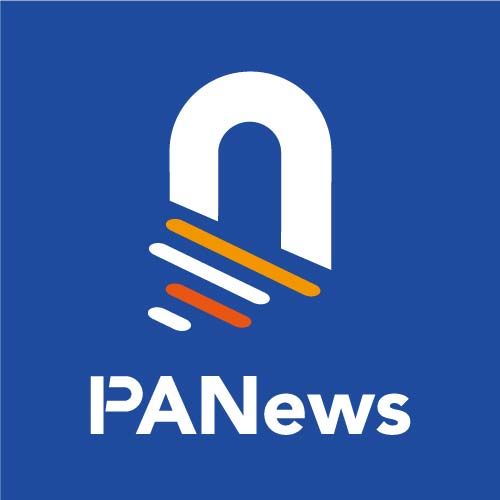
Reprinted from panewslab
03/28/2025·1MOriginal author: Elliot Hershberg
Compiled by: LlamaC
Text 👇
Ten years ago, Scientology believer (not a scientist) Bob Dugan sold the American biotech startup he founded to AbbVie for $21 billion (yes, not a million). His company Pharmacyclics has developed a promising anti-cancer drug and has received great rewards. In fact, Dugan's revenue of more than $3.5 billion was reported to be one of the most rewarding public acquisition cases in history.
Apart from the mavericks of founder Dugan - and the involvement of several other big names, making the entire incident a record-breaking - it was originally a relatively ordinary deal. A large pharmaceutical company is facing tremendous pressure to find a new source of revenue as the patent exclusive period for one of its blockbuster products is coming to an end. And a breakthrough drug from an American startup just fills the gap.
A large part of the biotech industry is built on this dynamic. Small startups have become the main source of innovation, and undertake most of the early clinical trials each year. Large pharmaceutical companies are acquiring these startups at a generous premium, continuing to replenish their product pipelines.
But things are changing. Ten years later, Dugan made a comeback. This time, he brought a new drug to beat Merck's $30 billion cancer immunotherapy drug Keytruda in clinical trials. The key is that Dugan did not discover the drug in a US laboratory, but obtained authorization from a Chinese company.
Unlike Pharmacyclics, the story doesn’t end with a sky-high acquisition of billions of dollars. Instead, Merck turned to the same source and purchased its own version of the same drug from another Chinese company for $500 million.
This is of great significance. As we have seen recently in the field of artificial intelligence, China has become a competitive threat that cannot be underestimated in the field of biotechnology, demonstrating the ability to rapidly develop new drugs that can compete with — or even surpass — with — American laboratory products. In other words, “the pharmaceutical industry is ushering in its ' deepseek moment’.”
Let me give you another example. With the explosive success of the GLP-1 class, pharmaceutical companies are competing to acquire their next generation of such products to compete with Novo Nordisk and Eli Lilly for market share. Merck once again turned his attention to China and acquired an oral GLP-1 drug with a prepayment of $112 million. The transaction also includes subsequent milestone payments based on commercial success, with a total of US$1.9 billion.
As a background, American biopharmaceutical company Viking Therapeutics has an oral GLP-1/GIP agonist in its R&D pipeline, with a market value of US$3.8 billion. Instead of a full acquisition of Viking, why not get a molecule from China at a low price to see if it works?
In a time of sluggish biotech markets, increased external competition has made the situation even more difficult. Although the M&A market has slowed down, founders and investors are now unable to sleep at night because of secret Chinese competitors they have never heard of at first-minute yellow deals.
The entire situation triggered a lot of analysis. By far, I appreciate the most are David Li, the founder of Chinese-American biotech startup, his follow-up analysis in Timmerman Report, Bloomberg’s report, and Alex Telford’s thoughtful insight on “Will all our drugs come from China?”
Here, I want to step back and think about how we got to this point.
In 1987, Merck was named "the most respected company in the United States" for its "betting on the magical elements".
In the decades before that, Merck scientists were responsible for the development of breakthrough molecules against high blood pressure, some of the most successful vaccines in history (Morris Hillerman, one of the most productive vaccine scientists in history, was a Merck scientist), the first statins, and a whole new category of antibiotics.
Today, for the two hottest products on the market, Merck is not relying on the American biotech startup ecosystem (which was still in a difficult start in 1987), but instead takes molecules from the Chinese ecosystem – until recently, China has become an important source of innovation.
Obviously, the global drug research and development industry has undergone significant evolution. At this juncture, I think it is necessary to think about the long process of commercialization of drug research and development technology and the impact of this historical model on the future of the industry.
The long journey of commercialization of drug research and development technology
Let's think about biologics.
In the long history, most drugs are plant-based chemicals discovered by humans by chance and have a positive impact on human physiology. Over time, tools have been developed that can screen chemical space more systematically for useful small molecules. Another subcategory is proteins such as insulin that can be isolated from animals and used to treat diseases.
Genentech's founding in 1976 was a revolutionary breakthrough in recombinant DNA technology, which sowed the seeds of radical change. With the help of genetic engineering tools, people can produce biogenic molecules in a completely new way to alleviate disease. Fifty years later, the number of biological agents approved each year has almost the same as that of small molecule drugs.
Biologics increase over time
But initially, few people believed that this new drug would become possible. Moreover, there are also very few people in the world who have the necessary skills to try. It’s easy to overlook the fact that Genentech started out as a marginal project, with scientists working day and night, wearing uniform T-shirts, jeans and running shoes.
As Peter Thiel said, the company holds an important common secret that the outside world has not yet understood.
Successfully changed all this. When Genentech achieved its first product breakthrough, the market responded with fanatical optimism. Nothing—even Princess Diana—is more exciting than transforming life.
When Genentech went public in 1980, the initial share price was $35. Just one hour after the trading began, the stock price soared to $88, almost tripling. The scene at that time was simply fanatical.
By 1983, U.S. companies had invested $500 million in emerging biotechnology companies. Two years later, the U.S. Department of Commerce estimates that about 200 biotech companies have attracted nearly $2 billion in investment in total.
Looking back at the past, it is obviously a hype bubble. Recombinant DNA technology was still in its infancy at the time. Major breakthroughs like recombinant insulin have not come quickly. Regulatory issues remain unresolved. Large-scale production of biological agents is also a challenge.
The initial prosperity is followed by a period of disillusionment and contraction. In 1985, a Massachusetts reporter wrote:
If all this seems too far away for scientists with little or no business record, then you are right. This alone is that in the post-prosperity biotech industry, two trends have dominated.
First, among a few more capital-rich companies, scientists/founders/CEOs are hiring, or forced to hire traditional "digital experts" as company presidents or chief operating officers. These are experienced business executives from large companies, not academics. Cambridge-based BioTechnica International has just hired a veteran who has worked for DuPont for 20 years. At Collaborative Research, near Lexington, the new president once led a subsidiary of Johnson & Johnson. The same is true of the new president of Damon Biotech in Niedham Heights.
Second, the industry momentum seems to have turned to favoring big companies. Especially those companies that provide a large amount of original funding for biotech startups through equity purchases and R&D contracts. In a sense, the good response from the IPO of new biotech companies is just delaying bad news for many companies. Now, when these young companies are in urgent need of a new round of capital injection, companies such as DuPont, Grace, Monsanto and Eli Lilly are turning their investments to internal biotechnology capabilities. Therefore, industry experts predict a wave of mergers and acquisitions. EF Hutton analyst Nelson Schneider believes that up to two-thirds of biotech companies are either merged or acquired by large pharmaceutical or chemical manufacturers.
In other words, the industry is forced to mature and truly realize revenue, and existing organizations are awakening and start building their own biotech capabilities internally. By the late 1980s, most biotech stocks had lost three-quarters of their value.
But at the heart of this bubble, there is an important core of truth: recombinant DNA technology is indeed a revolutionary tool for making new drugs. Even in a calmer environment, companies that have resources, technology, talents and perseverance to survive are constantly launching new products.
After insulin was synthesized, Genentech produced seven more biological agents throughout the 1980s and 1990s. Amgen is another early biologic pioneer founded in 1980, who stand out from the fierce competition with a range of breakthrough drugs. Regeneration Corporation was founded in 1988. After its initial prosperity, they gradually established their own characteristics through in-depth research on the human genome and a powerful monoclonal antibody production technology platform - monoclonal antibodies have proved to be one of the most important types of biological agents.
Although there are still many skeptics, the scope of application of biological products continues to expand in the medical field, and by 2022, its approved number is the same as that of small molecule drugs for the first time. (The chart we saw before.)
The commercial success of these pioneers is also undeniable. Genentech was acquired by Roche for US$46.8 billion in 2009 and is still operating as an influential independent subsidiary. Amgen's current market value is US$168 billion. Regeneration is currently valued at US$78 billion, with its share price rising nearly 4,000% from its initial public offering. 2
This evolution is almost a textbook example of the Gartner hype cycle. The initial "innovation trigger" triggered a huge wave of hype and excitement. When the speculation cannot be fulfilled immediately, the market will calm down. If the initial trigger point does have substantial content, a more gradual rebound will occur over time.
We do seem to have entered a "productive plateau". The ability to produce biological agents is no longer a secret that a few companies are strictly keeping. Scientists around the world have spent decades refining the tools to develop these drugs. A large number of companies have emerged like mushrooms after a rain to provide antibody development services.
Take Adimab as an example. Founded in 2007, the company utilizes a new generation of antibody engineering technology, namely yeast surface display technology, to rapidly produce new molecules for many partners. Currently, they have worked with more than a hundred different partners, "more than 75 clinical projects originated from our platform."
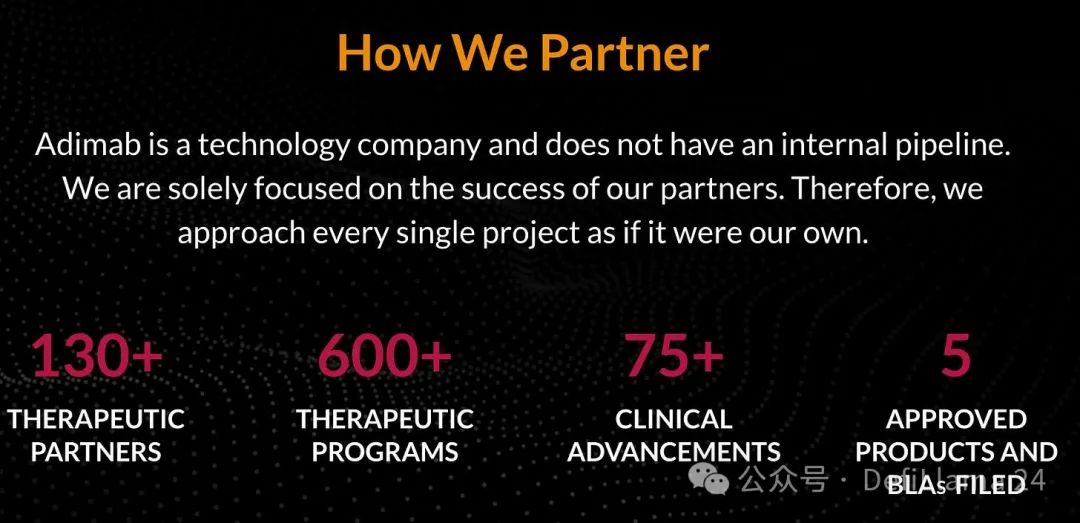 Source: Adimab
Given that Adimab is a private company, it is difficult to directly compare its commercial success with pioneers like Amgen or Regeneron. But based on the valuation of US$1.1 billion in a secondary transaction ten years ago, it has roughly grown into a company of approximately US$5 billion to US$10 billion.
Today, if companies want to adopt different technologies or have specific preferences in cost, speed, geographical location and even a variety of other factors, they can choose to work with FairJourney Biologics (valued at approximately US$900 million in 2024), OmniAb (capitalized at approximately US$400 million), Ablexis, Specifica, Creative Biolabs, Twist Biosciences, Alloy Therapeutics or other companies. This list is just an example and is far from exhausted all options.
It is worth noting that the size of each generation of enterprises has decreased by about an order of magnitude. The pioneers grew into companies over $100 billion, and then the rising leading service providers became companies over $10 billion, while new entrants to the market are companies over $1 billion.
In my opinion, this is like the definition of commodification in textbooks, the process of gradually converting goods or services into commodities and competing on prices. Commodities refer to goods or services that can be exchanged with other similar goods.
Think about electronics. Initially, only a few companies could produce the best TVs, which charged high premiums. Over time, this premium has been worn out by competition. Today, many companies sell large-size flat screens with smart features on Costco for hundreds of dollars. This is the process of commodification.
Similarly, differences among antibody discovery service providers are becoming increasingly difficult to distinguish, with many companies using similar technologies to produce antibodies against the same drug targets.
So far, we have only focused on the history of antibodies. But I want to ask you and me to assume boldly that this might annoy some drug developers: Nothing found to be immune to the inevitable trend of commodification—like almost every other technology.
For molecules of all sizes, once the technology is discovered — whether it is high-throughput screening, computer simulated screening, in vitro or in vivo models, or analytical detection — is standardized, companies around the world will compete to provide it as a service.
This is a long journey of modal commercialization.
Over time, revolutionary ideas became the universal cornerstone of the next wave of innovation.
Strategic evolution of biotechnology
While discovering the standardization and commercialization of technology, biotech investment is also becoming specialized. After decades of improvement, the industry tends to adopt standardized company valuation models and new strategies emerge.
One widely recognized strategy is the "fast follower" approach, which is to develop a new drug that makes it the "best of its kind" in the targets targeted by existing drugs, rather than targeting completely new drug targets as the "first".
An analysis published in 2003 pointed out two key advantages of “pursuing the best.” First, these drugs obviously have lower risk characteristics, because their targets have been verified by drug approval based on human evidence. Investors often discuss the extent of “biological risks” they assume for the new target hypothesis. Second, the risk difference does not appear to be rewarded accordingly. In fact, looking at drugs launched between 1991 and 2000, most blockbusters were developed for known targets, and fast followers create more value than risky innovative drugs.
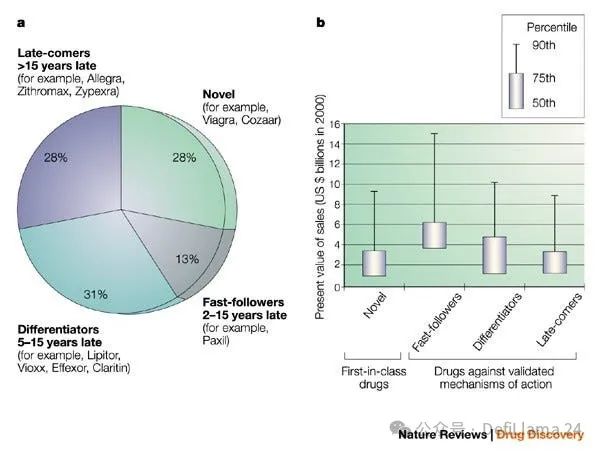 Pursuing the best
Merck's acquisition of the oral GLP-1 agonist we have studied before is a stark example of this strategy. Novo Nordisk and Eli Lilly took huge risks in verifying the efficacy of the first batch of GLP-1 drugs. Nowadays, other companies are racing to develop fast follow-up products with improved properties, such as replacement of injections in pills.
Many biotech investors have pushed such analysis to the extreme of logic. With the scale of early financing rounds and the expansion of funds behind them, it is increasingly difficult to justify the large amount of capital betting on completely unproven target hypothesis. In practice, this has led to serious clustering in the fields of proven targets.
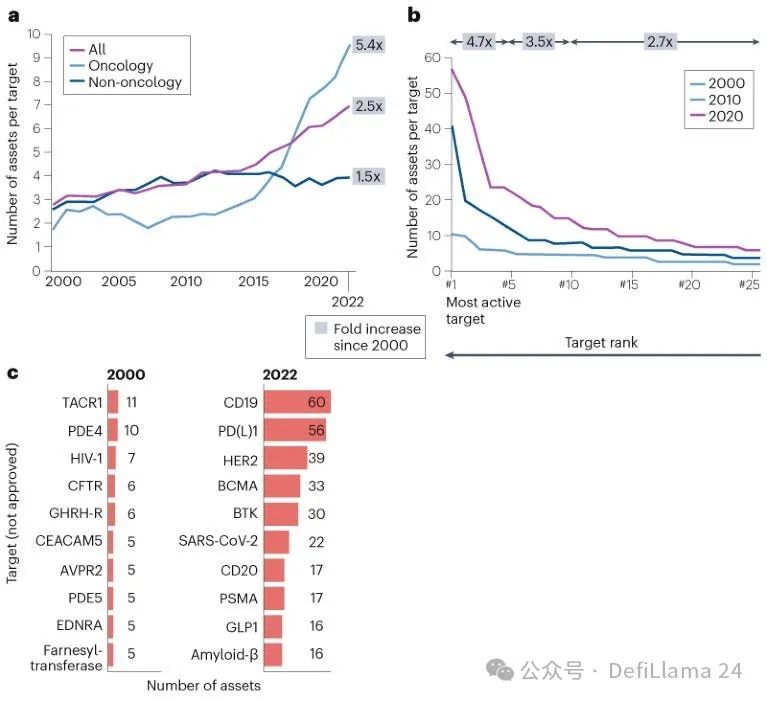 Aggregation phenomenon in drug development pipeline
To make things more efficient, “virtual biotech companies” emerged around the 2010s, outsourcing all R&D to discovery partners like Adimab. The goal is usually to quickly develop the best molecules targeting known targets and then sell them to large pharmaceutical companies for late development and commercialization.
This industry history is crucial to understanding the recent surge in China's licensing agreements, as many of the top outsourcing partners are contract research organizations (CROs) in China.
WuXi Biologics, a Chinese giant company that provides comprehensive biologic discovery and manufacturing services, has become the world's second largest outsourcing partner, accounting for more than 10% of the global market share.
Today, China's very logical strategic evolution reflected in its new policy in 2015 is to shift from a purely service provider to independent research and development of drugs. In a world where most people use standardized discovery technology to target the same drug targets, China has two key advantages:
speed. A new series of reforms enable clinical trials to be launched faster.
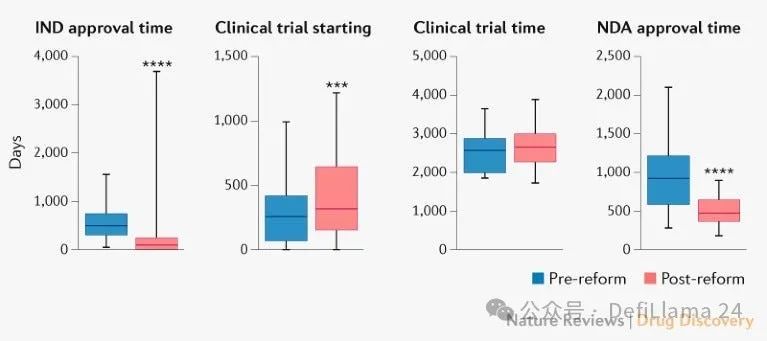 Trends in China\'s innovative drug research and development (Thanks again to Alex Telford\'s wonderful blog post to get me aware of this data!)
cost. Chinese scientists are paid only a small part of American scientists. A large army of highly skilled – often American trained – researchers can devote themselves to research on more problems.
With these advantages, Chinese startups and biopharmaceutical companies appear to have covered areas of known drug targets. Enterprises deploy cheap "call options" to a wide range of targets in the form of preclinical or early-stage assets. When a specific target or product concept is favored by large pharmaceutical companies, these "options" can be executed by increasing investment to accelerate the process of existing projects.
This puts huge pressure on the fast follow-up strategy. When American scientists fall asleep at night, machines in competitor labs on the other side of the planet are still buzzing.
To date, we have traced the history of commercialization of drug discovery technologies and the subsequent specialization of biotech investments. These changes help provide context for the industry’s “DeepSeek Moment.”
Now, let us think about areas where future value may accumulate.
Artificial intelligence may be the last wave of commodification
Over the past few years, a large amount of money has poured into companies with ambitions and aimed at using artificial intelligence to transform drug research and development. Companies like Xaira Therapeutics, which started with $1 billion in "seeds" funds, aim to independently develop drugs. But many companies, including EvolutionaryScale, Profluent, Chai Discovery and Latent Labs, prefer Adimab-like strategies to deliver the new technology as a widely-enabled infrastructure.
When Latent Labs was founded, Tony Kulesa of Pillar wrote:
This gave birth to a clear vision: popularize the use of advanced artificial intelligence tools in the field of drug discovery. Although every biotech and pharmaceutical company looking for therapeutic molecules understands the role of artificial intelligence, most companies do not have the ability to develop their own cutting-edge models and tools. Simon’s insight is that by giving partners instant access to the best tools, Latent Labs can accelerate the drug design process across the industry.
The combination of large-scale financing and new business models has triggered complex reactions that are both curious and skeptical. "The establishment of Latent shows how AI-centric startups can break traditions in biotech. Most biotech companies are established around a molecule, research paper or key intellectual property, while Latent investors bet on the AI talents of Kohl and another former DeepMind developer, AlphaFold's Alex Bridgland, and believe they can find a way out."
Let's consider the bear and bull market situations of this investment argument.
In a bear market, compared with commodity technology, these technical directions cannot significantly promote progress, whether they focus on new data generation, model expansion, architecture improvement, or a combination of these three.
In a live notes at the MIT Molecular Machine Learning Conference, Simon Barnet of Dimension wrote: “My understanding of the speech of [Adimab co-founder] Dr. Witrup, who believes that monoclonal antibodies (mAb) discovery is basically a solved problem, and the impact of machine learning (ML) on this field is exaggerated.”
If AI technology ultimately only has a small quantitative impact on issues such as antibody discovery, companies that provide these solutions may join the ranks of numerous companies competing for such services. We may see companies with a market capitalization of less than $1 billion, rather than generation giants with around $50 billion to $100 billion.
How is the bull market? Squint with me and imagine that the progress of AI will bring a qualitative leap that truly leads us into a world of design rather than discovery. Imagine a model that predicts platonic antibodies in zero samples—with perfect affinity and specificity for any target, and is extremely optimized in every dimension. Enter the Target Product Features (TPP) and you will get a drug.
That could be a big deal.
A frequently cited comparison object is Cadence Design Systems, a $66 billion company that generates a large portion of its revenue from licensing its Electronic Design Automation (EDA) software and intellectual property to the semiconductor industry. In high-value areas, the best design tools can be of great value. So, will the "Cadence of the Pharmaceutical Industry" be larger?
Is there any evidence to support this technological development trajectory?
Last March, the University of Washington's Baker Laboratory published a preprint paper titled "Designing Single-Domain Antibody with Atomic Accuracy." Based on decades of leading work in the field of computational protein design, they introduced an AI model that can effectively generate micro-antibodies (called VHHs or nano-antibodies) for specific targets.
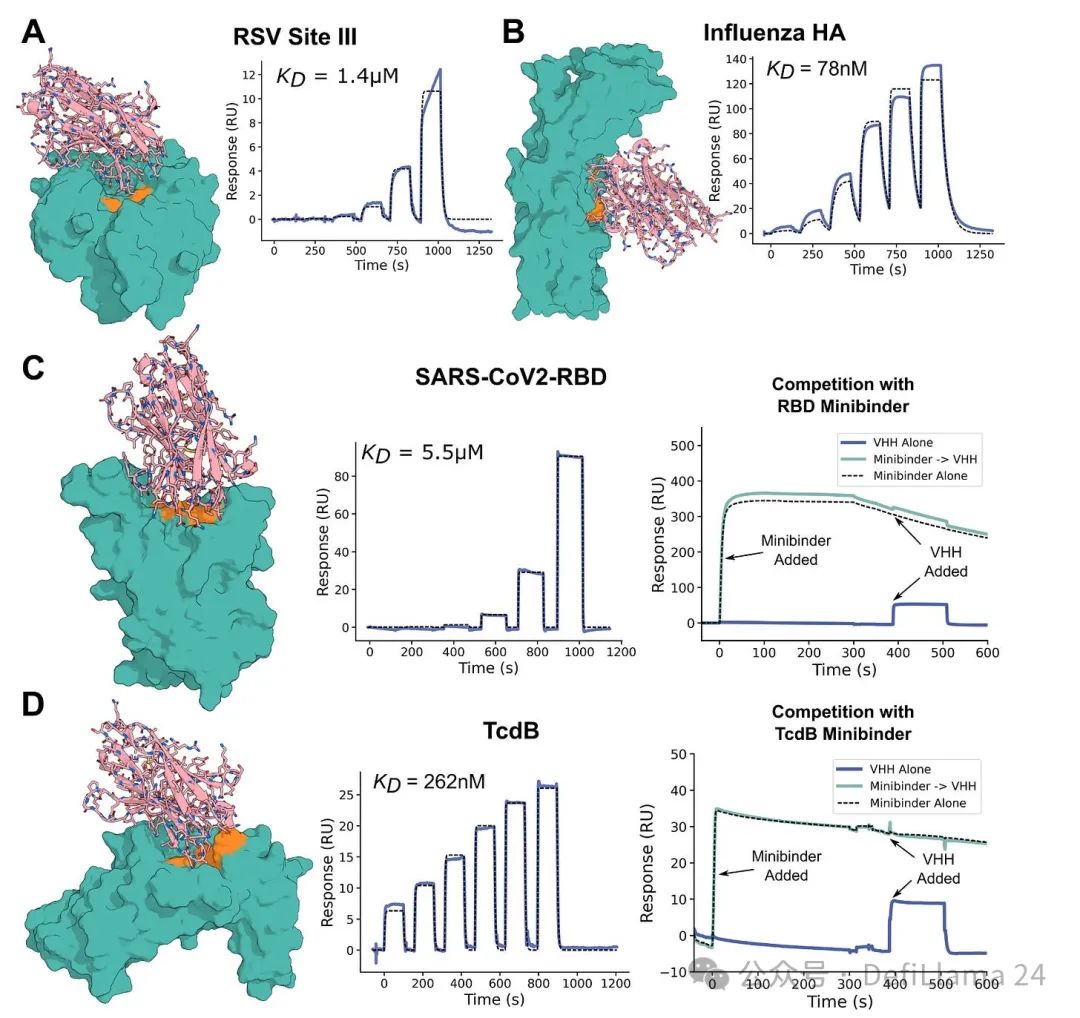 Figure 2 Part of the content: Atomic accuracy de novo design of single domain antibodies. The binding agent is shown in pink and the target protein is cyan green. The specified epitope (i.e., the region where the antibody binds) is displayed in orange.
These results sparked great excitement and interest—including a $1 billion bet invested to launch Xaira. But this work is just a proof of concept, not a magical black box that can spit out perfect antibodies. Scientists point out that the affinity of the produced nanobody to its target is still too weak to become a drug. And nano-antibodies are a strange protein that is not exactly similar to human antibodies—which again limits their utility in many clinical applications.
Less than a year later, Baker Labs "significantly updated" their initial preprint, renamed it "Decision of Atomically Accurate Antibody Design with RFdiffusion." As you might have guessed, the title changed because the scope of the study has been extended beyond the VHH design. The updated preprint also demonstrates the design of single-stranded variable fragments (scFvs), another form of antibody that has two variable domains, rather than a single variable domain of VHH.
Another important update is the response to affinity issues. "Although the affinity shown by the initial computational design is average, affinity maturation with OrthoRep can produce single-digit nanomolar binding that maintains the selectivity of a predetermined epitope," the author wrote. In other words, artificial intelligence cannot currently produce perfect combinations, but can be quickly adjusted and optimized through existing experimental techniques.
So it's about a year. At the risk of scribing between two data points, progress seems to be quite rapid. Looking ahead, what if someone used OrthoRep to create a huge affinity training dataset, and this step departed from the domain of atoms and encoded in the bit world in the form of improved model weights?
What will hinder the continued development from VHHs to scFvs to mature monoclonal antibodies in the next five years?
Again, we seem to be on the verge of digitalization of biologics development. If the advantages of speed, cost - and possibly quality - are significant enough, this could lead to the discovery market integration, and new entrants quickly attract a large share of outsourcing work, replacing existing businesses.
Now let us think carefully about what the world will look like under the concept of "basic model". What if the important basic models of biological structure and function are learned through numerous tasks? As Simon Kohl of Latent Labs said to Endpoints, “This vision is even more grand. I think we can expand on this and over time we will find that many other areas outside the molecular interaction level can also be guided by generative models.”
So if this point—or any part I just outlined—is true, some of these companies could become very large.
But one of the biggest threats is likely to be…commodification! After all, the entire "deepseek moment" framework originated from the sudden leap in AI capabilities with Chinese research teams having fewer resources than their American counterparts.
There are already signs of this.
To date, Demis Hassabis, co-winner of the Nobel Prize for Protein Structure Prediction and CEO of DeepMind and Isomorphic Labs, has been betting on algorithmic innovation rather than building proprietary data moats to ensure model defensiveness. In a recent interview, he said: "Make your algorithm better and your model better. You do have enough data - as long as you are innovative enough in your algorithm."
Surprisingly, serious algorithm competitors emerged so quickly.
In May 2024, Isomorphic and DeepMind published a paper introducing its latest and most advanced structural prediction model, AlphaFold3. In September of the same year, Chai Discovery released and open sourced a cutting-edge model. About two months later, a team of MIT research institutes launched another open source version with comparable performance.
In this new AI competition, how value will accumulate is worth paying attention to.
In any case, all these advances will open up new opportunities for innovation in drug development and other links.
Discovering the platform may become more valuable
Not all biotech platforms focus on therapeutic models. Some companies are focusing on the other side of the coin: identifying new biological targets to develop drugs. In Steve Holtzman's "Platform Companies Typology", these are called "Insight Platforms."
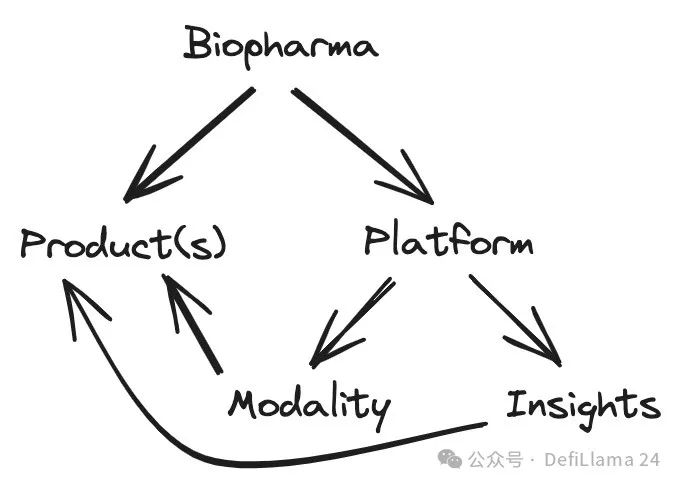 About Biotech Platform Strategy
Focusing on disease insights also comes with a range of strategic challenges. Holtzman wrote in his original post:
However, Class 2 A-type platform companies face challenges that a series of Class 1 platform companies have not encountered. These challenges are essentially due to the output of Class 2 A platform companies that are data/information/insight, rather than the production of new chemical entities (NCEs) and biotherapeutic drugs as Class 1 platform companies do.
- The data history of the biopharmaceutical industry is its commercialization history.
- Companies with drugs/products as their "lifeline" have a vested interest in "pre-competitive" data (or at least do so after being exclusive for a period of time). They win with their products; they are unwilling to be bound by information owners.
- The intellectual property (IP) environment is becoming increasingly strict: Gone are the days when a logical form of patent authorization (which can be antibodies, antisense nucleic acids, RNA interference, gene therapy, small molecules, etc.) can be obtained by simply displaying the transcriptional profile of a gene overexpressing (or gene mutations in a disease state) in a diseased tissue (or in any way) can be obtained.
- Furthermore, over time, the needs of the customer base have become more extensive. In the 1990s, most large pharmaceutical companies’ customers were willing to accept the terms that restricted data use to small molecule drug discovery and development (as this is the only area they are engaged in), and today, all pharmaceutical companies/large biotechnology companies require the right to utilize this data for all therapeutic mode development.
- Finally, Genus 2, Species A platform company has and builds its expertise in data generation, management, and analysis. It does not possess or cannot afford significant investment in drug discovery/development in one or more therapeutic modes, or in-depth biological/translational capacity development in one or more disease areas.
- Final result: Genus 2, Species A platform company abandoned its data/information business and established and became a drug discovery and development company.
Let's break it down.
First, it is important to realize that data generation technology itself has also gone through a long process of commodification. (That's technology, guys!) Second, the main problem in the past was asymmetric negotiations with large partners—they had exclusively discovered technology, which gave them an unfair advantage in actually creating chemicals against new targets.
This dynamic has begun to change. The growth and maturity of the CRO industry has enabled insight companies to engage in partner discussions with their own new chemical entities (NCEs) rather than simply patents centered around target insights.
What if artificial intelligence accelerates this dynamic? Over time, as the model becomes more commercialized, the time and cost of insights from targets to developing chemicals may be further compressed.
In this world order, the balance may tilt. New disease insights targeting known targets may exceed any incremental starting point in the chemical space.
After all, the success story of GLP-1 drug over $100 billion is built on biological insights rather than advances in technological models.
There are several economic and technological realities that may slow progress in this regard.
On the economic level, as David Yang pointed out, the reason why GLP-1 is a success story in the pharmaceutical industry rather than a victory for biotech companies is partly due to the centrality of M&A in the industry. Most early biotech investors are counting on realizing capital flows through large acquisitions, meaning they keep an eye on the list of acquisitions from the pharmaceutical giants. And pharmaceutical buyers are indeed reluctant to spend billions to test new biological assumptions—especially when the scale of market opportunities is still unclear.
How can we change this and unleash a new wave of more innovative drugs? We need to continue to shorten the time and cost of every link from discovery, development to commercialization.
Doing so will make early drug discovery more valuable.
Improving discovery and commercialization capabilities seem to be technical issues. Accelerating development may require new technology and regulatory reforms. Drawing on China's experience (changing ideas this time!) and studying its recent reform measures may provide a good starting point for the latter.
On a technical level, it is important to recognize that simulating human biology is a more difficult AI problem than simulating specific patterns. Consider two questions: Does my antibodies bind this target with higher affinity? What impact will the activation of GLP-1 receptor have on the entire human physiology? The tools in the laboratory to clearly answer the first question are already available. The answer to the second question can only be fully known after the first human trial, because our preclinical model is only a rough approximation of human biology.
More efficient simulation of human biology may require large amounts of data generation and ongoing progress in new AI paradigms.
Over time, addressing these economic and technological challenges could greatly reshape the biopharmaceutical industry and lead a wave of emerging commercial biotech companies advancing bold new therapies.
But in the long run, all these rapidly superposition technologies may also lead to a more thorough deviation from the traditional biotech business model.
The moat may be very different
In most industries, there are a variety of viable strategies to build a lasting competitive advantage. The classic 7 Powers framework proposed by Hamilton Helmer is designed to enumerate the most commonly adopted approaches.
The 7 major forces are:
- Economy of scale – a business whose unit cost decreases as output increases.
- Internet economy - a business model in which the value realized by customers increases as the user base expands.
- Reverse positioning - Enterprises adopt a new and superior business model, and existing companies cannot follow suit because of fear of their existing business being eaten up.
- Convert Cost – A business model in which customers expect to suffer more than the value they gain from switching to alternatives.
- Brand effect - Due to the influence of historical information, a company enjoys higher perceived value when providing objectively the same products or services.
- Trapped Resources – A company has priority access to a coveted resource that can independently increase value.
- Process Power – The organizational structure and activity combination of an enterprise can achieve lower costs and/or better products, and these advantages can only be matched by long-term investment.
At the risk of oversimplification, in the biopharmaceutical world, only two forces really matter. Large pharmaceutical companies benefit from economies of scale because they are able to share the costs of development and commercialization through revenue from their existing product portfolios. For biotech companies, basically the only real source of power is monopoly resources controlled in the form of new intellectual property (IP).
As Peter Drucker once wrote, “The pharmaceutical industry is an information industry.” The value of small molecule drugs has nothing to do with their physical form, which is almost worthless. The ability to charge the highest profit on any existing physical product is purely a function of intellectual property. Once the intellectual property expires, generic drug manufacturers can quickly step in and offer a significantly reduced price.
This is what Peter Korczynsky of RA Capital defines as the “biotechnical social contract”. Scientists and entrepreneurs receive rewards for patent exclusive rights for innovation. But this exclusivity is limited. Once expired, innovative drugs will become cheap goods that future generations can afford.
Source: The United States Social Contract with the Biopharmaceutical Industry
The hidden concern of commercialization is that this may soon become a social contract between China and the biopharmaceutical industry - as long as they can produce products of the same intellectual property at a lower cost and faster pace.
But what if there is a different way to establish defensive barriers in the field of biotechnology?
There are some early examples pointing to defensive orthogonal forms. For CAR-T therapy, a new form of medicine centered on transforming patients' own cells to eliminate cancer, it is difficult to predict what its generalization will take. In Korcinsky's book on the Social Contract of Biotechnology, he actually expressed concerns about it. Companies selling these drugs may gain moats from process power rather than monopoly resources surrounding intellectual property.
现在让我们随着时间的推移向前推进。
个性化癌症疫苗是另一种具有此类形态的治疗方法。这类药物的核心并非单一的化学成分构成。相反,每一剂都是通过患者测量数据、算法和生产步骤的复杂组合而制成。
这带来了非常有趣的后果。这可能是第一种具有网络经济效应的药物。由于每一剂药物都是通过算法设计的,随着收集的数据增多,其质量可以得到提升。患者可以从拥有最大数据壁垒的公司生产的药物中获益。这种方法显然也受益于流程力量。随着时间的推移,作为市场领导者,这种新模式的赢家甚至可能积累起明显的品牌优势。
如果更多形式的药物开始呈现这种趋势,我们可能会看到一波生物技术公司直接竞争,试图将自己确立为全新一代的制药公司。
想到这里,我不禁想起我的好友Packy McCormick 关于垂直整合者的论述。用他的话来说,这些公司有几个决定性特征:
垂直整合企业是指那些:
- 整合多项前沿且经过验证的技术。
- 在其技术栈中发展重要的内部能力。
- 模块化标准化组件,同时掌控整体系统集成。
- 直接与现有行业巨头竞争。
- 提供更优质、更快速或更经济的产品(通常三者兼具)。
对于垂直整合者而言,整合本身就是创新。
和以往一样,这一策略面临明显挑战。天下没有免费的午餐!
一个巨大的障碍很可能是融资和资本形成。这种公司创建方式与大多数生物技术投资者考虑产生回报的方式完全不同。目前完全不清楚大型制药公司是否愿意在没有明确商业可行性证明的情况下收购拥有如此复杂产品的公司。
该领域的赢家可能需要另寻资金来源。一个可行的选择是利用日益壮大的"深度科技"风险资本池,这类资本专注于支持硬件领域的突破性进展和原子世界的创新。后期投资可能来自综合性成长型股权公司,而非传统的生物科技跨界基金。
在生物技术领域尝试打造垂直整合者,绝非胆小者所能胜任。
以新方式融合多种技术实属不易。融资将充满挑战。扩大商业化努力同样艰难。成功可能需要远比预期更长的时间。
鉴于所有这些因素,生物技术投资可能开始反映私募市场的整体演变趋势。企业可能会延长私有化状态的时间。以SpaceX 为例,这家公司在私有化的23 年间筹集了近100 亿美元资金,目前估值已达3500 亿美元。早期投资者和员工的流动性主要来自二级市场,而非并购交易或首次公开募股。
尽管困难重重,潜在的回报却是巨大的。
曾经难以想象的测量工具如今在生物学领域已司空见惯。催生上一代生物技术浪潮的基础洞见已被精炼并商品化。人工智能正在加速生物学向预测性和定量学科的转变。
解决癌症、传染病和大脑健康等以往方法难以应对的重大问题,可能需要整合多种数字与物理构建模块的创新解决方案。
如果这些解决全球问题的公司以新方式建立护城河,我们可能会见证首批市值超过1 万亿美元的生物技术企业诞生。
当前公开生物技术市场的形势相当黯淡。对于美国生物技术公司而言,中国收购活动的持续升温进一步威胁了它们的成功前景。正如Adam Feuerstein 所写:“市场情绪糟糕透顶,悲观氛围挥之不去,以至于人们开始严肃质疑这个行业是否还有翻身的可能。”
与此同时,早期市场充满潜力。技术创新的步伐同样迅猛。那些汲取了宝贵经验并掌握强大工具的创业者们,正在追求全新的理念。
或许正确的问题不是市场是否会反弹。因为它终将反弹。市场是周期性的。相反,问题在于生物技术是否正处于向全新阶段转变的边缘。如果是这样,那么现在正是创业的最佳时机。
in other words……
生物科技已死。生物科技万岁!